Opportunity & Unrealized Potential
Manufacturing industries are failing to capitalize on the tremendous potential of artificial intelligence and machine learning. While some may blame the limits of technology, a dearth of data science talent, or the resistance of workers rooted in inertia, the root cause of the friction is a lack of data integrity.
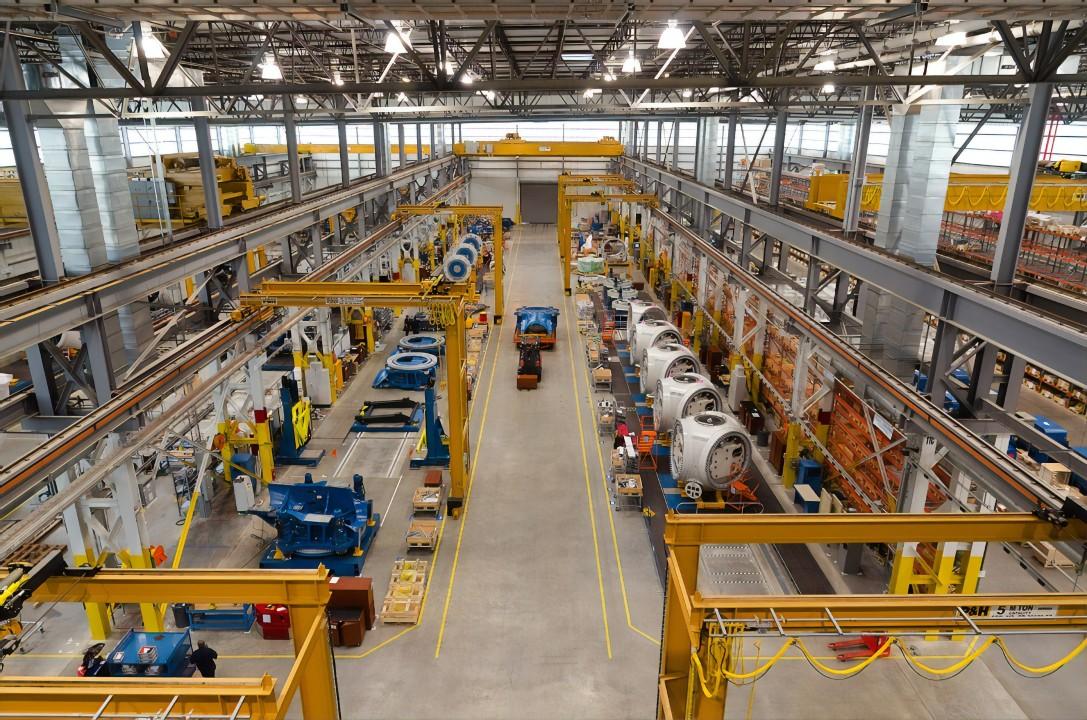
Most manufacturers’ IT infrastructures and data practices were established in a pre-AI era, not anticipating the value of insight and not built to deliver or optimize AI/ML-ready data. Thoughtful tactical approaches to data preparation and cleaning can address some of these issues. Scalable solutions, however, will require enterprise-level strategic change – in other words, the CEO and Board of Directors must give a clear mandate to establish data integrity and seize the opportunities it creates.
Accelerating Change from the Top Down
Unfortunately, manufacturing industries have yet to realize the benefits of optimized activity available from existing data — a late-to-the-game situation that has existential consequences given the state of global competition. The matrix-oriented organizational structure pervasive in the Global 2000, in which P&L responsibility for discrete business units creates ‘silos’ of activity, renders blind spots that mask and distract leaders from new areas of leverage and opportunity.
Operational Technology (OT), the term of art that refers to technology related to machines and production operations (contrasted with Information Technology (IT), which largely refers to the connectivity of people and the information they create and consume) sits squarely in a gaping blind spot of the corporate matrix. Workers and supervisors in a factory are focused on production and throughput using machines and related processes, while the IT department is focused on ensuring that the people in the factory can communicate, receive direction on process, and access relevant corporate information. Who is responsible for the technology related to the machines and the machine-level data pouring out every second? This is not clear, and its lack of clarity has resulted in no specific role in most companies accountable and incented to capture, harness, and deploy information and activity related to OT.
Process improvements have been the focus of productivity efforts for decades, and methodologies such as Kaizen and Six Sigma have become accepted standards to be globally competitive. The benefits to be gained from process improvements now are part of the status quo, yet there remain many hundreds of basis points of reliability and productivity improvements available for capture but not accessible through process improvements alone. This known but not-yet-captured opportunity for incremental profit and productivity is waiting for those who clearly establish and incentivize excellence in the area of OT and the data it surfaces. As a newly accessible opportunity in the blind spot of the matrix, action by corporate leaders (i.e. the CEO and the Board of Directors) is the definitive path to establish accountability for action and its resulting profit.
The magnitude of this opportunity, the confusion of the blind spot, and the clarity of profit from decisive action are the motives that led me to begin a movement of executives to advocate for a Board-level Data Integrity Committee at every organization in the Global 2000.
This board committee would oversee the activity of not only IT but also OT, governed by the mandate to both leverage and protect the company’s data for the benefit of shareholders, customers, and society at large. Oversight from the Data Integrity Committee would ensure that a company’s operational data is accurate, complete, and secure. It would protect sensitive consumer and business data from internal misuse, rogue hackers, and the threat of data theft by foreign state actors. The committee would hold leaders accountable for making data-driven decisions, encourage executives to leverage existing troves of enterprise data, and mine the treasures of newfound profit and defensible moats buried in the data.
Only upon a foundation of data integrity can AI fulfill its promise in manufacturing. Only then can machine learning turn data into meaningful, trusted intelligence. Only then will information empower manufacturing leaders to know the complete array of facts, ask the right questions, make decisions with total clarity, and see around corners. And only then will the industry not just survive amidst tremendous pressures, but thrive – creating goods, machines, and infrastructure that serve people around the globe.
Constraints on Success
The economic stakes for manufacturing AI are high. In December, the ISM manufacturing index for the U.S. registered its lowest level since the 2009 great recession, with only minor signs of growth in intervening months. In Germany, economic growth slumped to a six-year low, largely due to manufacturing sector challenges.
Amidst demand, legacy technology, and equipment companies, from Siemens to Intel to Bosch to Microsoft, are investing billions of dollars in aggregate to bring effective AI to the manufacturing sector. In parallel, purpose-built industrial AI software companies, like Uptake, are leveraging new technology capabilities to address this need. These providers deploy AI to enable manufacturers to predict failures before they happen, better manage maintenance costs, and increase Overall Equipment Effectiveness – all in an effort to drive profitability in a punishing business environment.
However, a recent Bain survey of 600 high-tech executives found that industrial customers were less optimistic about predictive maintenance than they were two years earlier. Implementing predictive solutions across maintenance, quality, and throughput has been more difficult than anticipated, and it has proven challenging to extract valuable insights from the data. This has led to a massive missed opportunity: There are billions of data points created by the machines and equipment in our manufacturing plants, but a shocking 1% of that data is used.
The Elephant in the Factory
To understand the dynamics behind these disappointing outcomes, it is useful to consider the situation from the perspective of a typical manufacturing executive. He likely has at most a surface-level understanding of how machine learning works:
- Collect some data
- Create some models
- Drive business results.
Soon after projects launch, however, leaders and their teams discover execution can be quite complicated. Often, it’s not the Data Science that is the issue; it is the data itself.
Industrial data tends to live in wildly disparate systems. Those systems don’t talk the same way or have the same labeling classifications. Time-series data has inconsistent timestamps. Data is frequently missing or filled with errors. The result is that a typical data scientist spends 80 percent of her time data wrangling – that is, cleaning, structuring, and enriching raw data so it is AI/ML-ready. At many large manufacturers, data collection and storage are so mismanaged that Data Science isn’t even possible.
These data integrity challenges are the elephant in the room – or the factory floor, to extend the metaphor. Data problems are a low priority for most manufacturing leaders, yet often the biggest barrier to AI-enabled success.
Challenges Collecting & Structuring Data
One major US automaker, a global leader in its industry, had invested in a massive technology infrastructure over the course of decades. However, it wasn’t designed around predictive maintenance, because AI-driven smart manufacturing wasn’t possible even five years ago. As a result, successive IT and OT leaders made a series of innocuous, yet costly mistakes.
The company was often failing to preserve data that would be valuable later. For example, they sought to predict failures of a robot arm at a factory based on sensor readings. At the time, operators were maintaining two years of work order data, rich information for predicting machine failures. However, they were only storing the prior 256 seconds of sensor readings. That’s because the system was designed to troubleshoot what happened during the 7 minutes just before a fault or failure – not predict what might be coming.
The fix was clear: Add a tag to a programmable logic controller (PLC) to historicize those readings and preserve it in a PI historian. Once they took that action, though, the team was essentially launching data collection from scratch. It would be months before they had enough data to develop useful machine learning models.
The same company was collecting much of its asset data reactively, not proactively. For example, they were collecting temperature readings on one factory machine, but not pressure data.
Given the history, the logic behind this practice seemed sound: The machine had years before experienced a failure, and the maintenance teams discovered the root cause was temperature. Consequently, they added a temperature tag, failing to take into account either physics of failure or the history of fault modes across this asset over time. Again, once they added the pressure channel, it would be months before they had enough data to do any exploratory data science incorporating that set of readings.
Unfortunately, even when the company was collecting and storing crucial data, it had failed to construct a common data structure across the enterprise. This meant that, even in a single factory, they were collecting data from machines and components with wholly different time stamps. For example, one might be collecting 100 Hz readings; the other monthly readings.
This led to time-intensive, complex data wrangling to harmonize and prepare data – a necessary step before building machine learning models to explore correlations among readings on those assets. In retrospect, that effort could have been avoided if, with an eye toward AI, they had developed a common data structure that can compare data apples-to-apples.
Issues in Monitoring Data & Ensuring Quality
Many manufacturers also make mistakes around monitoring data and ensuring the quality of data that is input into these systems.
The case of a major transportation equipment manufacturer is a cautionary tale. The data ingestion pipelines coming from the manufacturer’s IT systems to their AI software provider had been running dry for eight hours. The AI provider was the one to call the company’s manager, who had no idea; none of his colleagues had noticed either. There was no monitoring system for their machines to ensure data was continuously flowing. This lack of oversight meant that at any time, the insights from AI/ML models might not be trustworthy.
“Garbage in, garbage out” is a decades-old computer science cliché. The output of models won’t be accurate if the incoming data is a mess. Yet many manufacturers ignore this principle at their peril.
At one leading steel manufacturer, 60 percent of work order descriptions were classified by technicians as “Null.” This made it difficult for data scientists to connect asset signals to problems that would require maintenance. The poor data was partly a result of IT teams’ lack of thought ahead of time in configuring maintenance management systems. They included vague categories in the drop-downs, and no one was checking up on technicians to ensure high-quality data inputs.
This potentially rich data was left as tribal knowledge among some maintenance team members, instead of repeatably transformed into efficient AI models.
Employing AI to Clean Data
When data is messy, missing, unstructured, or not monitored well, AI can be used to clean data and fill gaps.
This approach helped a transcontinental, Class I freight railway that had limited visibility into its maintenance performance. The company was shopping locomotives for failures every month, far more than the regulated 90 days. Like every industrial business, it sought to transition operations from reactive to proactive. To make this happen, its leaders needed to gain greater insight into locomotive health – including unconnected systems that don’t have sensors and don’t transmit data – and the effectiveness of its repairs.
They saw potential in sorting and cost-analyzing millions of work order records by failure mode category – for example, a dynamic brake problem. They wanted to go further by inspecting technicians’ descriptions to pinpoint the root cause of failure – like ground relay, grid blower, or distributed power. They had already tried manual approaches to wrangling relevant data sets, but the speed, scale, and low quality of the data were too much to manage.
The team worked with a provider of AI-enabled label correction to turn uncategorized (or improperly categorized) work order data into accurately labeled data, based on a training set of high-quality data. For example, the process re-categorized unassigned failure codes into specific events like positive train control or event recorder communication. This flipped the data set from 43 percent unusable to 93 percent clean.
With a data set they could trust, the railway was able to strike an informed balance between low-risk, low-reward maintenance tasks and high-risk, high-reward maintenance tasks without incurring additional risk. They could then use data-driven recommendations to make the right repairs for each job. The team also established a closed feedback data loop to track how implemented maintenance practices contributed to improving asset health and performance.
This kind of AI-enabled data cleaning impacts operations long after the project is over. At one mining processor, technicians had been adding natural language information into an electronic maintenance database for 40 years. While the technicians undoubtedly used the system for their own working notes, the data wasn’t being used for broad analysis. Not surprisingly, it was filled with messy shorthand and gaps. After cleaning was instituted, the quality of their inputs increased markedly.
Part of that change might be explained by the Hawthorne effect: when people know they’re being watched, they behave better. Yet many of the technicians expressed excitement that their information was being used to feed AI/ML models, and they wanted to be helpful.
I believe the manufacturing industry could benefit from similar initiatives, borrowing from what has worked well among its industrial peers.
Early Indicators & Lessons from Fleet
While data cleaning efforts may be valuable, they are not sufficient for manufacturers to fully capitalize on Data Science. This is why the most forward-thinking manufacturers are investing to overhaul their infrastructure to ensure all corporate data is AI/ML-ready. Some are relying on internal expertise, while others are relying on the support of systems integrators or software companies.
One major agriculture equipment manufacturer is now maintaining relatable files across the enterprise for all of its key assets, with a single, central pivot table as a way to reference in and query. Others are taking honest inventories of their data, down to every dispersed legacy system and silo, to account for where all of it lives. Many are connecting the databases for their shop maintenance systems and operational asset failure systems together. The resulting data helps produce insights into which strategic problems would be worth a look – and ensures that data are always available to develop ML models to impact key KPIs.
These sweeping changes are finally enabling the smart manufacturing future we’ve been wishing for to emerge into reality: fixing problems before they happen; optimizing preventive maintenance schedules for performance and cost; increasing productivity without putting asset life at risk.
What might data integrity enable at scale throughout the sector? The global trucking industry presents an excellent case study. About 20 years ago, the Society of Automotive Engineers standardized fault codes under what is called SAE J1939. At the time, fault codes differed by manufacturer and model – even within fleets, maintenance record reports would use different codes. This meant widespread confusion among fleet management and maintenance teams.
After J1939, standard VRMS codes – which are sort of like a Dewey decimal system for trucks – made harmonizing component reporting, repair, and work orders simple. This has been a boon for AI in Fleet, with outcomes ranging from improved driver behavior to lower fuel costs.
Summary
For corporations to fully leverage AI and machine learning, there must be a commitment to data integrity. This commitment needs to occur at the board-level to ensure the company’s data is precise, thorough, and secure, ultimately allowing the organization to recognize the value of AI. Uptake is leading this charge and helping manufacturers harness and clean their data to increase the availability of assets, improve asset reliability, and streamline operations. The future of manufacturing relies on executive and board leadership taking a stand for the integrity of their company’s data and embracing the untapped power of AI.